Why Your Company Needs an Artificial Intelligence Product Manager
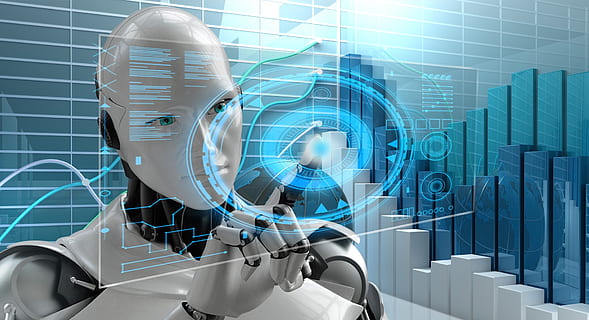
What is an Artificial Intelligence Product Manager anyways? Today’s business is complex and is rapidly evolving. Users expect more from businesses and companies are leveraging the user data to gather insights, solve complex business problems, and deliver solutions in ways that were not possible before. Businesses, both big and small are dipping their toes into AI and machine learning to deliver move value to their users and delight their customers.
AI Brings New Challanges
As more and more companies jump on the bandwagon to build their AI and machine learning capabilities, these companies often start by hiring or retraining engineers to kick start their AI efforts. When building AI products, the foundational way of software delivery changes. Data becomes the foundation of product development. This data are in different formats and repositories across the enterprise have to be integrated, cleaned, and pre-processed. These data are then fed into machine learning models to get the output, which is fed into the end-user product or is used to drive business processes.
Machine Learning Models are Sensitive
Machine learning models can be fragile. If the underlying data changes, which most likely they will, the model will drift. This drift will change the model behavior, giving wrong answers if the input data is not representative of real-world data. This in turn will break the business processes. Over time, the business will collect more data that could be added to the models, in which case the model will have to be retrained again. More likely than not, the model optimization and retraining will be ongoing rather than one time as the business and customer behavior changes. These challenges with AI/ machine learning are new to software development that businesses need to adapt to.
This is where an Artificial Intelligence Product Manager comes in. Product Managers sit at the intersection of business and technology to ensure that AI projects succeed. Here are the key areas where Product Managers can help businesses in the transition to AI and data-first company.
AI is Not a Silver Bullet
AI can solve a lot of problems for business but it is not the end all be all solution. In fact, there are many problems that can be and should be solved without AI. Product Managers can help determine what are the right business problems that can be solved with Machine Learning and what problems should be solved with other approaches like simple rule-based programs. Even when using AI and machine learning to solve a particular problem, Product Managers can determine the trade-offs such as choosing a model that has high accuracy but requires a lot of optimization vs one with a reasonable accuracy that requires much less machine learning effort. Artificial Intelligence Product Managers need to understand and make the business understand that AI is not a silver bullet and set reasonable expectations.
AI Centric Processes
Unlike software methodologies like Agile, there are no mature and systematic machine learning methodologies. Teams are often new to the technology as they are developing machine learning skills. Many of the development processes are immature. This is where Product Managers play a critical role in helping the business understand the complexities and changes that come with machine learning development environments. Creating the right set of processes and systems that work for data as well as traditional engineering teams is critical for machine learning products.
Explainability
AI can understand past data and deliver inferences and answers in a way that humans cannot. This sometimes results in situations where data scientists cannot explain why AI arrived at a certain decision. Even when decisions can be explained, it is not uncommon for the business teams to compare AI decisions to the manual way of doing things. A Product Manager can help the business stakeholders understand the nuances of AI. They can abstract the complex machine learning concepts to explain what features were used to train the models, how models operate, and why the results of the models can be trusted, even if the exact ways in which decision is made cannot be determined. They can act as a bridge to translate the machine learning process to help business position and explain the results to end customers.
Not Enough Data
Product Managers need to explain to the business why data they have is not enough to solve the problems business is looking to solve. They need to instill and change the AI mindset and educate the stakeholders from the get-go. Otherwise, the project will fail and not meet the expectations. Product Managers can also help in identifying the data that the company needs to collect in order to make machine learning possible. They can create data hooks within the product and help aggregate data from segregated sources both inside and outside the company to kick start the machine learning efforts.
Air Cover for Engineering Teams
A great Product Manager protects the data scientists and engineers from the business when results are not as expected. This is true for any product but it becomes even more critical for a machine learning project. Product Managers need to explain why the results can change over time, or why the behavior seems erratic. They need to set reasonable expectations for the business leaders, be patient because often you don’t know if a machine learning model will work at all. Even if it does, the roadmaps are difficult to set, and timelines are hard to lock down until you have tried the models.
AI Product Manager is a critical role in any organization that’s working with AI. What these companies need are more people who can speak the language of the business, understand AI technologies, and be comfortable with data. That is what a good AI Product Manager brings to the table. Without an AI Product Manager, companies will continue to experience a disconnect between business and AI implementations, and the general disappointment on a promise to deliver.
What is your company’s AI strategy? How are you building your team to kick start your machine learning efforts? I would love to hear about it.